Illustration Research
Illustrations pose an important image processing challenge. Not only are sketches sparse and noisy, but there exists a large diversity of drawing styles. Furthermore, very precise results are required for real-world usage scenarios. The research here focuses on tackling these problems to aid illustrators in the content creation process.
-
Smart Inker
We present an interactive approach for inking, which is the process of turning a pencil rough sketch into a clean line drawing. The approach, which we call the Smart Inker, consists of several “smart” tools that intuitively react to user input, while guided by the input rough sketch, to efficiently and naturally connect lines, erase shading, and fine-tune the line drawing output. Our approach is data-driven: the tools are based on fully convolutional networks, which we train to exploit both the user edits and inaccurate rough sketch to produce accurate line drawings, allowing high-performance interactive editing in real-time on a variety of challenging rough sketch images. For the training of the tools, we developed two key techniques: one is the creation of training data by simulation of vague and quick user edits; the other is a line normalization based on learning from vector data. These techniques, in combination with our sketch-specific data augmentation, allow us to train the tools on heterogeneous data without actual user interaction. We validate our approach with an in-depth user study, comparing it with professional illustration software, and show that our approach is able to reduce inking time by a factor of 1.8x while improving the results of amateur users.
-
Mastering Sketching
We present an integral framework for training sketch simplification networks that convert challenging rough sketches into clean line drawings. Our approach augments a simplification network with a discriminator network, training both networks jointly so that the discriminator network discerns whether a line drawing is a real training data or the output of the simplification network, which in turn tries to fool it. This approach has two major advantages: first, because the discriminator network learns the structure in line drawings, it encourages the output sketches of the simplification network to be more similar in appearance to the training sketches. Second, we can also train the networks with additional unsupervised data: by adding rough sketches and line drawings that are not corresponding to each other, we can improve the quality of the sketch simplification. Thanks to a difference in the architecture, our approach has advantages over similar adversarial training approaches in stability of training and the aforementioned ability to utilize unsupervised training data. We show how our framework can be used to train models that significantly outperform the state of the art in the sketch simplification task, despite using the same architecture for inference. We additionally present an approach to optimize for a single image, which improves accuracy at the cost of additional computation time. Finally, we show that, using the same framework, it is possible to train the network to perform the inverse problem, i.e., convert simple line sketches into pencil drawings, which is not possible using the standard mean squared error loss.
-
Sketch Simplification
We present a novel technique to simplify sketch drawings based on learning a series of convolution operators. In contrast to existing approaches that require vector images as input, we allow the more general and challenging input of rough raster sketches such as those obtained from scanning pencil sketches. We convert the rough sketch into a simplified version which is then amendable for vectorization. This is all done in a fully automatic way without user intervention. Our model consists of a fully convolutional neural network which, unlike most existing convolutional neural networks, is able to process images of any dimensions and aspect ratio as input, and outputs a simplified sketch which has the same dimensions as the input image. In order to teach our model to simplify, we present a new dataset of pairs of rough and simplified sketch drawings. By leveraging convolution operators in combination with efficient use of our proposed dataset, we are able to train our sketch simplification model. Our approach naturally overcomes the limitations of existing methods, e.g., vector images as input and long computation time; and we show that meaningful simplifications can be obtained for many different test cases. Finally, we validate our results with a user study in which we greatly outperform similar approaches and establish the state of the art in sketch simplification of raster images.
Publications
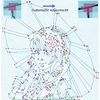
@Article{ChuanSIGGRAPH2024, author = {Chuan Yan and Yong Li and Deepali Aneja and Matthew Fisher and Edgar Simo-Serra and Yotam Gingold}, title = {{Deep Sketch Vectorization via Implicit Surface Extraction}}, journal = "ACM Transactions on Graphics (SIGGRAPH)", year = 2024, volume = 43, number = 4, }
@Article{MadonoPG2023, author = {Koki Madono and Edgar Simo-Serra}, title = {{Data-Driven Ink Painting Brushstroke Rendering}}, journal = {Computer Graphics Forum (Pacific Graphics)}, year = 2023, }
@InProceedings{CarrilloCVPRW2023, author = {Hernan Carrillo and Micha\"el Cl/'ement and Aur\'elie Bugeau and Edgar Simo-Serra}, title = {{Diffusart: Enhancing Line Art Colorization with Conditional Diffusion Models}}, booktitle = "Proceedings of the Conference on Computer Vision and Pattern Recognition Workshops (CVPRW)", year = 2023, }
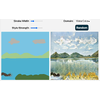
@Article{HuangCVM2023, title = {{Controllable Multi-domain Semantic Artwork Synthesis}}, author = {Yuantian Huang and Satoshi Iizuka and Edgar Simo-Serra and Kazuhiro Fukui}, journal = "Computational Visual Media", year = 2023, volume = 39, number = 2, }
@Article{HaoranSIGGRAPH2021, author = {Haoran Mo and Edgar Simo-Serra and Chengying Gao and Changqing Zou and Ruomei Wang}, title = {{General Virtual Sketching Framework for Vector Line Art}}, journal = "ACM Transactions on Graphics (SIGGRAPH)", year = 2021, volume = 40, number = 4, }
@InProceedings{ZhangCVPR2021, author = {Lvmin Zhang and Chengze Li and Edgar Simo-Serra and Yi Ji and Tien-Tsin Wong and Chunping Liu}, title = {{User-Guided Line Art Flat Filling with Split Filling Mechanism}}, booktitle = "Proceedings of the Conference on Computer Vision and Pattern Recognition (CVPR)", year = 2021, }
@InProceedings{YuanCVPRW2021, author = {Mingcheng Yuan and Edgar Simo-Serra}, title = {{Line Art Colorization with Concatenated Spatial Attention}}, booktitle = "Proceedings of the Conference on Computer Vision and Pattern Recognition Workshops (CVPRW)", year = 2021, }
Our algorithm is content-aware, with generated lighting effects naturally adapting to image structures, and can be used as an interactive tool to simplify current labor-intensive workflows for generating lighting effects for digital and matte paintings. In addition, our algorithm can also produce usable lighting effects for photographs or 3D rendered images. We evaluate our approach with both an in-depth qualitative and a quantitative analysis which includes a perceptual user study. Results show that our proposed approach is not only able to produce favorable lighting effects with respect to existing approaches, but also that it is able to significantly reduce the needed interaction time.
@Article{ZhangTOG2020, author = {Lvmin Zhang and Edgar Simo-Serra and Yi Ji and Chunping Liu}, title = {{Generating Digital Painting Lighting Effects via RGB-space Geometry}}, journal = "Transactions on Graphics (Presented at SIGGRAPH)", year = 2020, volume = 39, number = 2, }
@Article{SimoSerraSIGGRAPH2018, author = {Edgar Simo-Serra and Satoshi Iizuka and Hiroshi Ishikawa}, title = {{Real-Time Data-Driven Interactive Rough Sketch Inking}}, journal = "ACM Transactions on Graphics (SIGGRAPH)", year = 2018, volume = 37, number = 4, }
@Article{SasakiCGI2018, author = {Sasaki Kazuma and Satoshi Iizuka and Edgar Simo-Serra and Hiroshi Ishikawa}}, title = {{Learning to Restore Deteriorated Line Drawing}}, journal = "The Visual Computer (Proc. of Computer Graphics International)", year = {2018}, volume = {34}, pages = {1077--1085}, }
@Article{SimoSerraTOG2018, author = {Edgar Simo-Serra and Satoshi Iizuka and Hiroshi Ishikawa}, title = {{Mastering Sketching: Adversarial Augmentation for Structured Prediction}}, journal = "Transactions on Graphics (Presented at SIGGRAPH)", year = 2018, volume = 37, number = 1, }
@InProceedings{SasakiCVPR2017, author = {Kazuma Sasaki Satoshi Iizuka and Edgar Simo-Serra and Hiroshi Ishikawa}, title = {{Joint Gap Detection and Inpainting of Line Drawings}}, booktitle = "Proceedings of the Conference on Computer Vision and Pattern Recognition (CVPR)", year = 2017, }
@Article{SimoSerraSIGGRAPH2016, author = {Edgar Simo-Serra and Satoshi Iizuka and Kazuma Sasaki and Hiroshi Ishikawa}, title = {{Learning to Simplify: Fully Convolutional Networks for Rough Sketch Cleanup}}, journal = "ACM Transactions on Graphics (SIGGRAPH)", year = 2016, volume = 35, number = 4, }